Searh results
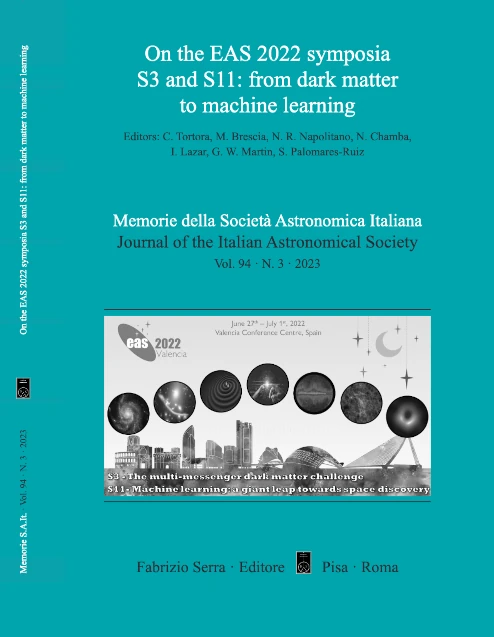
From Dark Matter to Machine Learning: A collection of EAS 2022 proceedings from the S3 and S11 symposia
Volume 94, n. 3, 2023
Index | |
Foreword |
S3: The dark matter multi-messenger challenge
S. Cebrián | Direct Detection of Dark Matter DOI: https://doi.org/10.36116/MEMSAIT_94N3.2023.11 |
Raghuveer Garani | Stellar probes of dark matter DOI: https://doi.org/10.36116/MEMSAIT_94N3.2023.17 |
Sisk-Reynes et al. | Current and Future constraints on Very-Light Axion-Like Particles from X-ray observations of cluster-hosted Active Galaxies DOI: https://doi.org/10.36116/MEMSAIT_94N3.2023.26 |
Gauri Sharma and Jonathan Freundlich | Dark Matter Halos of Disk-like Galaxies at z ∼ 1 DOI: https://doi.org/10.36116/MEMSAIT_94N3.2023.33 |
L. Ciotti | Rotation curves of galaxies in General Relativity DOI: https://doi.org/10.36116/MEMSAIT_94N3.2023.40 |
Bílek et al. | Imprint of the galactic acceleration scale on globular cluster systems: Galaxies in the Fornax Cluster DOI: https://doi.org/10.36116/MEMSAIT_94N3.2023.44 |
Lucas M. Valenzuela | Lights in the Dark: Globular clusters as dark matter tracers DOI: https://doi.org/10.36116/MEMSAIT_94N3.2023.47 |
Oliver Manzanilla Carretero, Adriana Bariego-Quintana, and Felipe J. Llanes-Estrada | Dark Matter Cigars DOI: https://doi.org/10.36116/MEMSAIT_94N3.2023.52 |
Marcel S. Pawlowski | What new observations tell us about Planes of Satellite Galaxies DOI: https://doi.org/10.36116/MEMSAIT_94N3.2023.55 |
Lucas C. Kimmig | And Yet There is Mass: How Projection Effects Can Solve the Apparent Lack of Mass in Substructures of Simulated Galaxy Clusters DOI: https://doi.org/10.36116/MEMSAIT_94N3.2023.60 |
G. Granata et al. | Investigating the discrepancy in sub-halo compactness between observed and simulated galaxy clusters with improved strong lensing modelling DOI: https://doi.org/10.36116/MEMSAIT_94N3.2023.63 |
M. Meneghetti et al. | Too many galaxy-galaxy strong lenses observed in galaxy clusters DOI: https://doi.org/10.36116/MEMSAIT_94N3.2023.67 |
Nemani et al. | Reconstructing blended galaxies with Machine Learning DOI: https://doi.org/10.36116/MEMSAIT_94N3.2023.111 |